KEYNOTE SPEAKERS
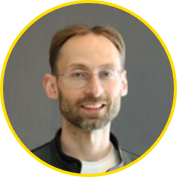
Michael BRONSTEIN
University of Oxford, UK
This keynote is sponsored by Applied Network Science, Springer
Physics-inspired Graph Neural Networks
Michael Bronstein is the DeepMind Professor of AI at the University of Oxford and Head of Graph Learning Research at Twitter. He was previously a professor at Imperial College London and held visiting appointments at Stanford, MIT, and Harvard, and has also been affiliated with three Institutes for Advanced Study (at TUM as a Rudolf Diesel Fellow (2017-2019), at Harvard as a Radcliffe fellow (2017-2018), and at Princeton as a short-time scholar (2020)). Michael received his PhD from the Technion in 2007. He is the recipient of the Royal Society Wolfson Research Merit Award, Royal Academy of Engineering Silver Medal, five ERC grants, two Google Faculty Research Awards, and two Amazon AWS ML Research Awards. He is a Member of the Academia Europaea, Fellow of IEEE, IAPR, BCS, and ELLIS, ACM Distinguished Speaker, and World Economic Forum Young Scientist. In addition to his academic career, Michael is a serial entrepreneur and founder of multiple startup companies, including Novafora, Invision (acquired by Intel in 2012), Videocites, and Fabula AI (acquired by Twitter in 2019).
-
The message-passing paradigm has been the “battle horse” of deep learning on graphs for several years, making graph neural networks a big success in a wide range of applications, from particle physics to protein design. From a theoretical viewpoint, it established the link to the Weisfeiler-Lehman hierarchy, allowing to analyse the expressive power of GNNs. We argue that the very “node-and-edge”-centric mindset of current graph deep learning schemes may hinder future progress in the field. As an alternative, we propose physics-inspired “continuous” learning models that open up a new trove of tools from the fields of differential geometry, algebraic topology, and differential equations so far largely unexplored in graph ML.
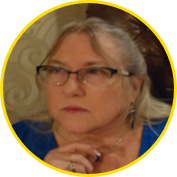
Kathleen M. CARLEY
Carnegie Mellon University, USA
Coupling in High Dimensional Networks
Kathleen M. Carley has a H.D. from the University of Zurich in Business, Economics and Informatics; a Ph.D. from Harvard University in Sociology; and two S.B.’s from Massachusetts Institute of Technology – one in Political Science and on in Economics. She is a professor of Societal Computing in the Software and Social Systems Department in the School of Computer Science with secondary appointments in the Departments of Electrical and Computer Engineering, Engineering and Public Policy, and the Social and Decision Sciences and in the Heinz School of Public Policy at Carnegie Mellon University. She is the director of two university wide centers: the Center for Computational Analysis of Social and Organizational Systems (CASOS) that focuses on the application of network science and other data science techniques to the study of complex issues such as diffusion and terrorism, and the Center for Informed Democracy and Social-cybersecurity (IDeaS) that focuses on online harms such as disinformation, hate-speech, and extremism. She is also the CEO of Netanomics.
Dr. Carley’s research combines cognitive science, social networks and computer science to address complex social and organizational problems. Specific research areas are dynamic network analysis, computational social and organization theory, social adaptation and evolution, and social-cybersecurity. Carley and her students have developed infrastructure tools for analyzing large-scale dynamic networks and various agent-based simulation systems. The infrastructure tools include: ORA, a statistical toolkit for analyzing and visualizing high dimensional networks in general and through time and space. NetMapper - a text-mining system for extracting semantic networks from texts, sentiment, cognitive cues, and other content. Her simulation models include: Construct – which is a general model for information and belief diffusion within and across media, and OSIRIS – which is a simplified digital twin of an organization undergoing various types of cyber attacks.
Dr. Carley is an IEEE fellow. She has served on numerous national academies panels. In addition she has received multiple awards including the Simmel award from INSNA, the United States Geospatial Intelligence Foundation Academic Award.
-
From an ecological perspective people and their networks adapt and link to other networks all of which co-evolve form dynamic high-dimensional networks. High dimensional networks are ones with one or more types of nodes (multi-modal) and one or more types of links (multi-plex) where there are a set of networks linked together by sharing at least one node. People are constrained and enabled by their position in these high dimensional systems, and are impacted by the whole not just by one network at a time. For example, in organizations people are embedded in both a social network of who knows who, a knowledge network of who knows what, an assignment network of who is doing what, a task requirements network of what knowledge is needed to do which task, and so forth. Today, high dimensional networks are easier to collect due to digital data and novel computational techniques for extracting networks from texts. Importantly, all these networks are coupled. As is classically the case for complex systems, a little coupling is valuable and a very high degree can lead to problems if not catastrophes. In this talk the power of high dimensional networks for addressing social issues and considerations that need to be addressed in new algorithms are discussed. It is argued that high dimensional networks often provide more explanatory power and enable prediction in cases where a social network alone does not, and that the co-evolution of the networks must be tracked to assess impact. New techniques that take such high dimensionality into account are described.
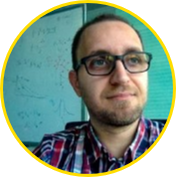
Manlio DE DOMENICO
University of Padua, Italy
This keynote is sponsored by PLOS
An Emerging Framework for the Functional Analysis of Complex Interconnected Systems
Manlio De Domenico is an associate Professor of Applied Physics and Head of the Complex Multilayer Networks (CoMuNe) Lab at the Department of Physics and Astronomy 'Galileo Galilei' of the University of Padua.
His research activity is at the edge of theoretical, experimental and computational aspects of statistical physics of complex systems, where theory is used to make hypothesis about empirical phenomena in biological, ecological, socio-technical and socio-ecological sciences, which are then validated on real (sometimes massive) data sets. To date, he has applied such tools to:
- The interactome of human and several other organisms
- The human, macaque and C. Elegans connectomes
- A variety of socio-ecological and socio-technical ecosystems
- The Internet and the Dark Web
- A variety of transportation infrastructures, including the global airport network, rail networks, road networks and multimodal urban transportation means
A (non-exhaustive) list of his current activities includes:
- The mathematical formulation of multiplex networks, the study of their structure and of dynamical processes on such systems, the study of their resilience to random or targeted pertubations
- The formulation of an appropriate statistical physics/information theory of complex networks
- The formulation of a geometry of network-driven processes
- The application of advanced mathematical techniques to reduce the complexity of networked systems
- The functional representation of a system from the measurement of signals produced by its units, with application to human brain, human interactome, climate change and social systems
He also finds enough time to investigate hidden structural and dynamical patterns in complex real and virtual time-varying networks, with particular attention to social, biological and economic systems. Indeed, he develops models and simulations for human mobility, the spreading of epidemics and of information in real-world social networks.
-
Information exchange is crucial for the functioning of interconnected systems, influencing -- and being influenced by -- the interplay between the underlying structure and dynamical rules in action. Analyzing empirical complex networks is challenging due to this interdependence.
I will present a framework -- combining statistical physics and information theory -- shifting from a structure-based approach to a functional analysis perspective. It has been successfully applied for inference problems and model selection, quantify the structural and functional (dis)similarity of networks, identify emergent functional modules, renormalize heterogeneous networks, cluster layers and reduce the dimensionality of multilayer systems.
Specifically, I will focus on a few applications of practical interest: (i) reducing multilayer and high-order systems; (ii) identifying units critical for information propagation; (iii) showing that ubiquitous topological features, such as modularity and small-worldness, emerge to optimize a trade-off for middle- to large-scale information exchange between system's units.
I will discuss how this framework can help us to enhance our understanding and design of biological, social and engineering systems.
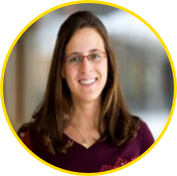
Danai KOUTRA
University of Michigan, USA
This keynote is sponsored by MDPI Entropy
Advances in Graph Neural Networks: Heterophily and Beyond
Danai Koutra is an Associate Professor in Computer Science and Engineering at the University of Michigan, where she leads the Graph Exploration and Mining at Scale (GEMS) Lab. She is also an Amazon Scholar. Her research focuses on principled, practical, and scalable methods for large-scale real networks, and her interests include graph learning, graph neural networks, graph summarization, knowledge graph mining, graph learning, similarity and alignment, and anomaly detection. She has won an NSF CAREER award, an ARO Young Investigator award, the 2020 SIGKDD Rising Star Award, research faculty awards from Google, Amazon, Facebook and Adobe, a Precision Health Investigator award, the 2016 ACM SIGKDD Dissertation award, and an honorable mention for the SCS Doctoral Dissertation Award (CMU). She holds a patent on bipartite graph alignment, and has multiple papers in top data mining conferences, including 9 award-winning papers and the 2022 IEEE ICDM Test-of-Time Award. She is Program co-Chair for ECML/PKDD 2023 and an Associate Editor of ACM TKDD. She was a track co-chair for The Web Conference 2022, a co-chair of the Deep Learning Day at KDD 2022, the Secretary of the new SIAG on Data Science in 2021, and has also routinely served in the organizing committees of all the major data mining conferences. She has worked at IBM, Microsoft Research, and Technicolor Research. She earned her Ph.D. and M.S. in Computer Science from CMU, and her diploma in Electrical and Computer Engineering at the National Technical University of Athens.
-
Graph learning, which leverages the complex relationships between entities in non-Euclidean data, has a wide range of high-impact applications, including recommendation systems, bioinformatics, fraud detection, and more. Graph neural networks (GNNs) have become one of the most popular graph deep learning models, achieving state-of-the-art results for semi-supervised classification, in which the goal is to infer the unknown labels of the nodes given partially labeled networks with node features. While many different GNN models have been proposed, most of them perform best in graphs that exhibit the property of homophily, sometimes referred to as the idea that “birds of a feather flock together”, in which linked nodes often belong to the same class or have similar features. However, in the real world, there are also many settings where “opposites attract”, leading to networks that exhibit heterophily, in which linked nodes tend to be from different classes. Many of the most popular GNNs fail to generalize well to networks with heterophily (low homophily). In this talk, I will present my group’s work on identifying effective GNN designs and introducing new architectures that can improve performance in heterophilous networks. I will also discuss connections between heterophily and other well-known challenges of GNNs, including oversmoothing, robustness, performance discrepancies across nodes, and scalability.
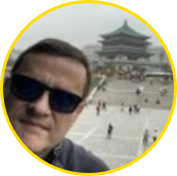
Romualdo PASTOR-SATORRAS
University Politècnica de Catalunya, Spain
This keynote is sponsored by MDPI Entropy
Opinion Depolarization in Interdependent Topics and the Effects of Heterogeneous Social Interactions
Romualdo Pastor-Satorras is full Professor at the Universitat Politècnica de Catalunya; He received a Ph.D. in Condensed Matter Physics from the Universitat de Barcelona in 1995. He spent four years as a postdoctoral researcher at the Massachusetts Institute of Technology (1996-1998) and The Abdus Salam International Centre for Theoretical Physics, ICTP (1998-2000). He has been a visiting scientist at Yale University (USA), the University of Notre Dame (USA), the Kavli Institute for Theoretical Physics (USA), the Helsinki University of Technology TKK (Finland), Indiana University (USA), and the Institute for Scientific Interchange (ISI) Foundation (Italy). He has been awarded twice with the national “ICREA Academia Prize” by the Government of Catalonia. He has published in more than 180 peer-reviewed journals in statistical physics. The main topics he works on are 1) Topological and temporal properties of natural systems. 2) Dynamical processes and non-equilibrium phase transitions in disordered substrates. 3) Dynamics of social systems. 4) Human activity and dynamics. 5) Non-Markovian temporal networks. 6) Collective motion.
-
The presence of opinion polarization (i.e. two groups holding opposite and possibly extreme opinions in a population) has been extensively observed with respect to several controversial topics, ranging from religion to political ideology. Modeling the process of reducing opinion polarization among the population, or depolarization, has been the object of much recent work. In most cases, such efforts address the simplest case of one-dimensional opinions with respect to a single topic. However, the process of opinion formation may invest multiple topics at the same time, requiring a proper multidimensional modeling framework for opinion dynamics. Here we present an analytically tractable model of opinion dynamics in a space of two interdependent topics, the so-called "Social Compass Model" (SCM). In the SCM, opinions are represented in polar coordinates, where the angle represents the orientation and the radius the conviction of individuals. We postulate a dynamics inspired by the classic Friedkin-Johnsen, in which the orientation of individuals, subject to an initial, preferred orientation and to social influence by peers, experience a depolarization phase transition for a sufficiently large social influence level. By means of a mean field analysis, we observe that the transition of the SCM is continuous for correlated initial opinions, while it has an discontinuous, explosive nature for uncorrelated initial opinions. These results are checked against numerical simulations using as initial opinions real data extracted form the American Nation Election Studies (ANES) surveys. Finally we discuss the effects of an heterogeneous pattern of contacts on the depolarization transition of the SCM model.

Tao ZHOU
USTC China
This keynote is sponsored by Applied Network Science, Springer
Recent Debates in Link Prediction
Tao Zhou is the founding director of the Big Data Research Center at the University of Electronic Science and Technology of China. His main research interests include network science (e.g., link prediction, influential node identification, epidemic spreading, etc.) and computational socioeconomics. He has published many research articles in prestigious journals (e.g., Physics Reports, PNAS, Nature Communication, PRL, etc.), which received >34000 citations from Google Scholar, with H-index=86. His works have been reported by many academic medias as Nature News, PNAS News, MIT Technology Review, Sci. Am., PhysOrg.com, My Science, TG Daily, Dutch Science Magazine, Chinese Science News, etc.
-
Link prediction is a paradigmatic problem in network science, which aims at estimating the existence likelihoods of non-observed links, based on known topology. This talk will briefly introduce three recent debates on link prediction. (1) Do machine learning models, including ensemble learning techniques, performs better than mechanism algorithms? (2) Which metric(s) can best evaluate algorithm performance: AUC, AUPR, Precision or others? (3) Are the shorter paths more important in link prediction than longer paths? For example, do 2-hop-based indices perform better than 3-hop-based indices?